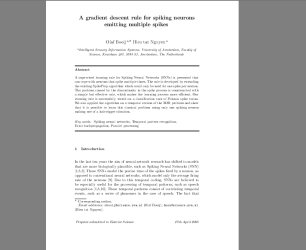
A gradient descent rule for spiking neurons emitting multiple spikes
Olaf Booij and Hieu tat Nguyen
Information Processing Letters, Volume 95, Issue 6, 30 September 2005, Pages 552-558. Special issue on spiking neural networks.
Abstract
A supervised learning rule for Spiking Neural Networks (SNNs) is presented that can cope with neurons that spike multiple times. The rule is developed by extending the existing SpikeProp algorithm which could only be used for one spike per neuron. The problem caused by the discontinuity in the spike process is counteracted with a simple but effective rule, which makes the learning process more efficient. Our learning rule is successfully tested on a classification task of Poisson spike trains. We also applied the algorithm on a temporal version of the XOR problem and show that it is possible to learn this classical problem using only one spiking neuron making use of a hairtrigger situation.
Downloads
Final version from science-direct for only $39.95 (I wonder how many articles elsevier sells...), or ask me for a free illegal version.
Preprint version: gzipped postscript (136 Kb), pdf (129 Kb).